How to tackle online survey fraud in market research: strategies and solutions
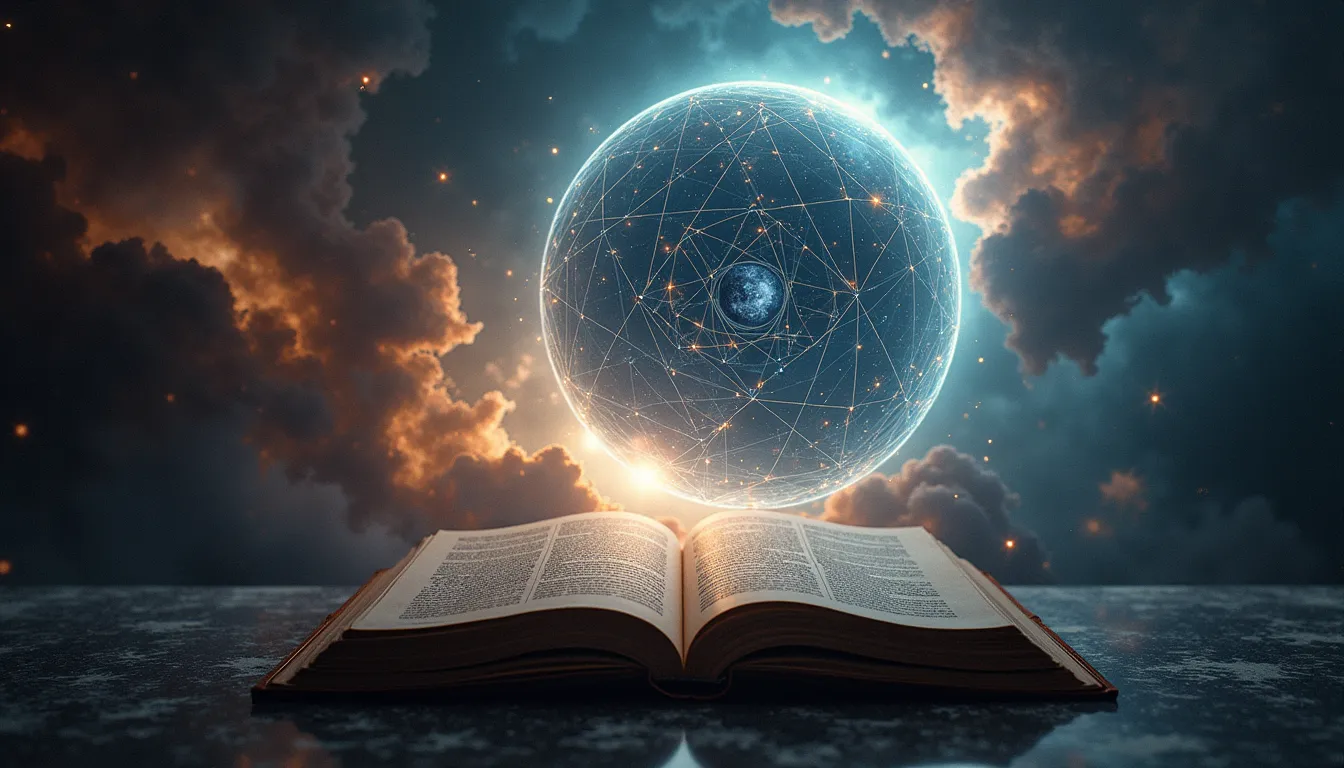
Online survey frauds in market research challenges and solutions present major challenges, leading to inaccurate data and poor decision-making. While online research is cost efficient, allowing for larger sample sizes and financial advantages, it faces challenges like fraud from participants, which can compromise research quality and budgets. In this article, we explore the types of survey frauds, their impact on research, and practical solutions to detect and prevent them. Learn how to safeguard your data’s integrity and ensure reliable market insights.
Key takeaways
- Understanding various types of online survey fraud, such as participant misrepresentation, bot responses, and multiple entries from the same IP address, is essential for effective data integrity management.
- The impact of online survey fraud extends to significant operational costs, compromised data integrity, and erosion of trust in market research methodologies, necessitating robust detection and prevention strategies.
- Implementing advanced detection methods such as AI, machine learning, and real-time risk scoring systems can significantly enhance the ability to identify and mitigate survey fraud, ensuring the reliability of collected data.
Types of online survey frauds
Online survey fraud manifests in various forms, each posing unique challenges to data integrity. Participant misrepresentation, where individuals provide false information to qualify for more surveys, is a common issue. Sophisticated fraudsters use various methods to avoid detection, such as using proxy servers to mask IP addresses and changing names or emails. Automated bot responses have surged, particularly since the pandemic, leading to a rise in fraudulent data.
Additionally, multiple entries from the same IP address are often used by participants to gain incentives unfairly, further skewing survey results. Understanding these types of fraud is crucial for implementing effective detection and prevention strategies.
Participant misrepresentation
Participant misrepresentation is a prevalent form of online survey fraud where respondents provide false or misleading demographic information to qualify for as many surveys as possible or maximize incentives. This fraudulent behavior results in skewed data, rendering the conclusions drawn from surveys unreliable. For instance, fraudulent participants might claim to belong to a specific age group or income bracket to fit the survey criteria, thereby contaminating the data pool with false data.
Combating participant misrepresentation involves using verification methods. Techniques such as cross-referencing demographic information with external databases and using advanced analytical tools can help identify and filter out fake responses.
Additionally, developing a framework for ethical participation and fostering a culture of honesty among survey participants can significantly reduce the incidence of this type of fraud.
Bot responses
The advent of automated bots has introduced a new dimension of online survey fraud. Bots are programmed to fill out surveys automatically, often leading to multiple and inconsistent responses that undermine the accuracy of collected data. These fake responses can be challenging to detect as bots can simulate human behavior.
However, techniques such as CAPTCHA tests and monitoring response patterns can help identify and mitigate the impact of bot responses on survey quality.
Multiple entries from same IP address
Multiple entries from the same IP address are a common tactic used by fraudulent participants to gain more incentives. This behavior leads to duplicate responses and skews the data, compromising the reliability of survey results. To combat this, companies like Kantar employ technology that prevents multiple submissions from the same IP address, thus preserving data integrity.
Additionally, tracking IP addresses and using digital fingerprinting techniques can help detect and prevent multiple submissions from the same source.
Emerging trends in survey fraud techniques
The landscape of survey fraud continues to evolve rapidly, with fraudsters developing increasingly sophisticated methods to bypass traditional detection systems. Understanding these emerging trends is crucial for staying ahead of potential threats to data integrity.
AI-generated fraudulent responses
The rise of advanced language models has introduced a new challenge in the form of AI-generated survey responses. These systems can create convincingly human-like answers that are difficult to distinguish from legitimate responses. Unlike bot responses of the past, which often contained obvious patterns or inconsistencies, AI-generated responses can appear thoughtful and contextually appropriate.
Research by the Market Research Society found that AI-generated fraudulent responses increased by 43% in 2023, with many passing through standard quality checks undetected. Organizations must now implement more advanced semantic analysis tools to identify these sophisticated forgeries.
Identity farming
A growing concern in the survey industry is "identity farming" – the practice of creating and maintaining multiple legitimate-seeming online personas over extended periods. Fraudsters invest time in developing these personas, completing profile questions consistently, and building response histories that appear authentic.
These cultivated identities are particularly problematic because they can pass panel quality measures designed to catch more opportunistic fraud. Identity farming operations often maintain detailed databases of persona information to ensure consistency across multiple surveys and platforms.
Collaboration networks
Survey fraudsters increasingly operate in organized networks, sharing information about qualification criteria, screening questions, and successful deception techniques. These collaboration networks utilize encrypted messaging platforms and private forums to coordinate their efforts, maximizing success rates and incentive collection.
A recent investigation identified over 30 active online communities dedicated to sharing survey qualification tips, with some having membership exceeding 5,000 participants. These networks can rapidly disseminate information about new surveys, allowing members to prepare convincing responses that match target demographics.
Geolocation spoofing
As geographic targeting becomes more important in market research, fraudsters have responded with increasingly sophisticated geolocation spoofing techniques. Advanced VPN and proxy services now allow participants to appear as if they're responding from specific targeted locations with high levels of precision.
Beyond simple country-level spoofing, these tools can now mimic specific cities, neighborhoods, or even facility locations, defeating basic IP-based verification methods. This trend particularly impacts location-based market research and requires multi-factor verification approaches to ensure geographic authenticity.
Hybrid human-bot approaches
Perhaps the most sophisticated emerging trend is the combination of automated tools with strategic human intervention. These hybrid approaches use automation to complete routine sections of surveys while alerting human operators when encountering verification challenges, open-ended questions, or other elements that might trigger fraud detection.
This methodology creates a particularly challenging pattern to detect, as the responses contain authentic human elements combined with the efficiency of automated completion. Detecting these hybrid approaches requires multidimensional analysis that examines both response patterns and content quality across different question types.
The impact of online survey fraud on market research
The repercussions of online survey fraud extend far beyond distorted data. Fraudulent responses can lead to misguided business decisions, wasting valuable resources and impacting company margins. Financial losses from the need to clean and validate compromised data further strain operational budgets.
Moreover, the presence of survey fraud erodes trust in market research methodologies, deterring companies from relying on online research and affecting the overall quality of market insights.
Data integrity issues
Survey fraud significantly undermines the integrity of research data. To combat this, it is crucial to ensure data quality through proactive measures aimed at detecting and preventing fraud. This poses a serious threat to the overall validity of research outcomes. Fraudulent responses lead to inaccuracies, affecting the conclusions drawn from surveys and risking decisions based on bad data. For instance, high rates of panel fraud can cause researchers to discard a substantial portion of collected data, with up to 38% deemed unreliable. This undermines the validity and reliability of findings, compromising overall research integrity.
Inadequate data-cleaning measures exacerbate these issues, potentially doubling the occurrence of survey fraud within a year. Robust quality checks and advanced validation techniques are essential to protect data integrity and ensure the accuracy of research outcomes.
Increased operational costs
The financial implications of survey fraud are substantial. Fraudulent responses necessitate additional resources for identification and cleaning, inflating market research expenses. The increased operational costs associated with overcoming survey fraud present a significant challenge for ensuring effective research results. As companies allocate more resources to validate data, the overall cost efficiency of market research is compromised.
Erosion of trust
Repeated occurrences of survey fraud lead to a fundamental mistrust in the methodologies utilized within market research. Continuous incidents erode trust in online survey methodologies and market research firms over time, deterring companies from utilizing these constantly evolving valuable tools, as highlighted by survey researchers.
Maintaining trust is critical, as the erosion of confidence in survey methodologies can significantly affect the overall quality of market research outcomes.
Detecting online survey fraud
Detecting online survey fraud is crucial for maintaining the integrity of survey data. Advanced detection methods, including automated quality assurance checks, IP tracking, and digital fingerprinting, play a vital role in identifying fraudulent activities. These techniques help ensure that data collected from online surveys is reliable and accurate, ultimately enhancing the quality of market research results.
IP tracking and digital fingerprinting
Tracking the IP address of the computer used to complete a survey is a fundamental method for identifying multiple submissions from the same source. By monitoring IP addresses and gathering relevant computer information, researchers can detect patterns indicative of fraud, such as multiple entries from the same IP address. Additionally, using phone numbers for validation can help verify participant information during registration and follow-up. However, legitimate shared computers can complicate tracking, making IP tracking not always reliable for repeated submissions.
Digital fingerprinting provides a more accurate detection method by distinguishing between devices. This technique, combined with the use of cookies to track user activity, helps identify duplicate submissions more effectively. To enhance this process, you may need to enable cookies.
Researchers can also employ time completion analysis and other check techniques to verify valid entries from the same IP address, ensuring the integrity of survey data.
Consistency checks and time analysis
Inconsistent answers in surveys often signal potential fraud. Analyzing response patterns and completion times can reveal abnormalities that might suggest disengagement or automated submissions. For example, unusually fast completion times may indicate bot activity, while inconsistent responses and multiple responses serve as a warning signal for fraudulent behavior and suspicious data.
These methods are crucial for maintaining data integrity and ensuring the quality of research outcomes.
Cross-verification techniques
Cross-verifying participant data with external sources, such as social media profiles, is an essential strategy for enhancing data reliability. Utilizing other records, like public databases and online platforms, can further validate participant information and prevent fraud. By validating participant information through cross-reference checks, researchers can significantly improve the authenticity of survey data. This method helps filter out fraudulent responses and ensures that the collected data accurately represents the target population.
Preventing online survey fraud
Preventing online survey fraud requires a proactive approach that includes implementing internal controls, fostering a culture of ethics, and employing advanced verification techniques as part of effective prevention techniques. Effective fraud prevention strategies not only enhance data accuracy but also reduce operational costs and maintain trust in market research methodologies.
Screening questions and trap questions
Screening questions are designed to identify and filter out ineligible participants who do not meet specific survey criteria, thereby ensuring that only eligible participants contribute to the data. Trap questions, on the other hand, are strategically placed to detect dishonest responses. For example, a trap question might ask for a specific detail that can be cross-checked with previous answers, addressing important research questions.
These techniques are crucial for maintaining survey quality and improving the reliability of collected data while also helping to collect data through qualitative interviews and conduct surveys.
CAPTCHA and incentive controls
CAPTCHA tests are effective in deterring bots from completing surveys, thus reducing the incidence of automated fraudulent responses. Additionally, controlling incentives can help mitigate fraudulent behavior. While lower incentives may reduce fraud, they could also decrease participation rates.
Balancing monetary and non-monetary incentives is essential to maintain participant interest without encouraging dishonesty. Informing participants about the consequences of fraudulent behavior can also deter potential fraudsters.
Random sampling and education
Random sampling is a crucial technique in survey research, as it enhances the representativeness of survey results and reduces biases. However, researchers face challenges in identifying and tracking potential participants, especially when using internet cookies. Effective random sampling helps combat the impact of survey fraud by ensuring a diverse and accurate sample of participants.
Educating participants about the importance of providing accurate and honest responses further improves data quality. Implementing educational initiatives helps participants understand their role in the research process, fostering better engagement and integrity.
Ethical considerations in combating survey fraud
Combating survey fraud presents significant ethical dilemmas for researchers. Balancing the need for accurate data collection with the privacy rights of participants is a challenging task. Researchers must ensure that fraud detection measures do not compromise participant confidentiality while maintaining the integrity of survey data.
Addressing these ethical considerations and ethical issues is essential for fostering trust and transparency in survey research.
Balancing detection and privacy
Implementing fraud detection measures can inadvertently compromise participant confidentiality, creating ethical dilemmas for researchers. Research ethics committees often encounter challenges in creating guidelines that effectively balance fraud detection and the privacy of participants.
Ensuring participant privacy while detecting fraud requires careful consideration of data handling practices to protect confidentiality and data security as part of ethical practices.
Informed consent and transparency
Obtaining informed consent is a cornerstone of ethical research practices. Researchers must clearly communicate how data will be used and shared, fostering trust among participants. Transparency in research practices not only builds participant trust but also enhances the integrity of the data collected.
By ensuring that participants understand the purpose and scope of the research, researchers can maintain ethical standards and encourage honest participation.
Fair compensation practices
Establishing fair compensation information for participants is vital for mitigating fraudulent behavior while encouraging genuine engagement in surveys. Implementing fair compensation strategies ensures that participants feel valued for their contributions and reduces the incentive for fraudulent behavior.
By offering equitable compensation, researchers can foster a more honest and engaged participant pool, ultimately improving the quality of survey data.
Advanced tools and technologies for fraud detection
The fight against online survey fraud has been significantly bolstered by advanced tools and new tools and technologies. These innovations are essential for improving data quality and preventing fraudulent activities. Market researchers now have access to artificial intelligence, machine learning, and other sophisticated systems that can detect and mitigate fraud in real-time, ensuring the integrity of survey data.
Artificial intelligence and machine learning
Artificial intelligence (AI) plays a crucial role in detecting fraudulent patterns by analyzing response data in real-time. Tracking online participants is essential to ensure survey integrity, as it helps identify participants accessing surveys from various browsers and monitor multiple attempts to participate. Machine learning algorithms can evaluate the likelihood of fraudulent activity as respondents complete surveys, providing immediate insights into potential fraud. These automated quality assurance checks can flag suspicious behavior before the data is analyzed, ensuring higher data integrity. The integration of AI and machine learning in survey methodologies significantly enhances the capability to prevent and detect fraud.
For instance, AI tools can identify anomalies in response patterns that are consistent with fraudulent behavior. This proactive approach allows researchers to address issues promptly, maintaining the highest quality of data. As these technologies continue to evolve, their ability to combat fraud will only improve, making them indispensable in the realm of market research.
Real-time risk scoring systems
Real-time risk scoring systems assess transaction details, user behaviors, and other factors to identify potentially fraudulent activities. These systems utilize algorithms that analyze responses and behaviors to determine the risk level of each survey participant.
By evaluating the potential for fraudulent behavior as surveys are completed, real-time risk scoring enhances the detection of anomalies and helps ensure the reliability of collected data.
Automated quality assurance checks
Automated quality assurance checks are critical in identifying potential fraud and ensuring data integrity before analysis. AI and machine learning algorithms can analyze patterns in survey responses to detect anomalies consistent with fraudulent behavior. These systems flag any suspicious responses for further investigation, enhancing the reliability of data collected from online surveys.
Implementing these tools ultimately restores trust in market research outcomes by ensuring the highest quality of data.
Implementing a multi-layered defense strategy against survey fraud
Effective fraud prevention requires a comprehensive approach that combines technological solutions with sound methodological practices. A multi-layered defense strategy provides redundant protection and adapts to evolving threats.
Risk assessment process
The foundation of any effective fraud prevention strategy begins with a thorough assessment of vulnerabilities in your current research methodology. This process identifies potential weak points and prioritizes improvements based on risk level and impact.
A comprehensive risk assessment should include:
- Audit of current fraud detection methods and their effectiveness
- Analysis of historical fraud patterns in your specific research areas
- Identification of high-value targets in your research program
- Evaluation of the potential impact of different types of fraud
- Prioritization of risk mitigation efforts based on vulnerability and consequence
Organizations should conduct formal risk assessments annually and brief reviews quarterly to adapt to emerging threats and changing research priorities.
Technology stack integration
Rather than relying on a single solution, effective fraud prevention requires the integration of complementary tools into a unified system. This approach creates multiple detection layers without creating unnecessary redundancy or friction.
Key considerations for technology integration include:
- Combining rule-based systems with AI/ML detection models
- Implementing real-time and post-hoc detection methods
- Balancing automated and human review processes
- Ensuring interoperability between different system components
A well-designed technology stack might include digital fingerprinting for identity verification, AI-powered response analysis, automated consistency checking, and human review of flagged responses—all working together through a central management platform.
Human review protocols
While technology plays a crucial role in fraud detection, human judgment remains essential for interpreting ambiguous cases and adapting to new fraud techniques. Establishing efficient workflows for manual verification prevents bottlenecks while maintaining data quality.
Effective human review protocols include:
- Clear guidelines for escalation and decision-making
- Tiered review processes based on risk levels
- Training programs to ensure consistent evaluation
- Workload management to prevent reviewer fatigue
- Documentation requirements for audit trails
Organizations should aim for a review process that examines approximately 10-15% of responses, with higher scrutiny applied to high-stakes research or studies with elevated fraud risk indicators.
Continuous improvement framework
Fraud prevention is not a static endeavor but requires ongoing adaptation to address evolving threats. A structured continuous improvement process ensures that your defenses remain effective against new fraud techniques.
Key elements of a continuous improvement framework include:
- Regular review of false positives and missed fraud cases
- Root cause analysis of significant breaches
- Performance tracking of various detection methods
- Systematic testing of system effectiveness
- Implementation of improvements based on findings
Leading research organizations typically operate on 90-day improvement cycles, with formal system evaluations and updates conducted quarterly to maintain defensive effectiveness.
Cost-benefit analysis model
Determining the appropriate level of investment in fraud prevention requires balancing the costs of implementation against the benefits of improved data quality. A structured decision-making framework helps optimize this balance.
An effective cost-benefit analysis should consider:
- Direct costs of fraud prevention tools and processes
- Operational impact on research timelines and participant experience
- Financial impact of potential data quality issues
- Reputational risk of decisions based on compromised data
- Competitive advantage of superior data quality
Organizations typically find optimal results by investing 3-5% of their total research budget in fraud prevention measures, though this may vary based on industry, research type, and specific risk factors.
By implementing this comprehensive, multi-layered approach to fraud prevention, market researchers can significantly reduce the impact of fraudulent responses while maintaining efficient operations and positive participant experiences.
Conclusion
Online survey fraud significantly threatens market research by skewing data, inflating costs, and eroding trust. Combatting this requires a proactive approach using advanced detection methods like AI and real-time risk scoring, alongside practical prevention techniques such as trap questions and IP tracking. Ethical considerations must balance data integrity with participant privacy. By staying vigilant and integrating these strategies, researchers can safeguard data reliability and ensure the accuracy of market insights.